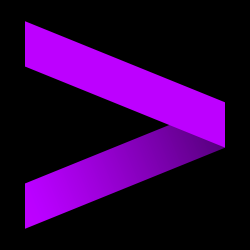
Accenture Finance & Risk Blog
The Accenture Finance & Risk portal of English language updates and articles has been decommissioned. Please watch out for a new series of regulatory and compliance updates and articles. To find out more about Accenture Digital Risk & Compliance please click here.